In this case study, we hear from Akira Murakami from English Language and Linguistics, who has been making use of BlueBEAR to enable his research into understanding how people acquire languages other than their native languages.
My research lies at the crossroads of second language acquisition (SLA) and corpus linguistics. SLA is a discipline devoted to understanding how people acquire languages other than their native languages. In my work, I harness large-scale text data (called corpora), such as collections of written pieces by second language (L2) learners, to delve into the intricacies of this process. Given the computational intensity of these investigations, I rely on BlueBEAR’s high-performance computing (HPC) service.
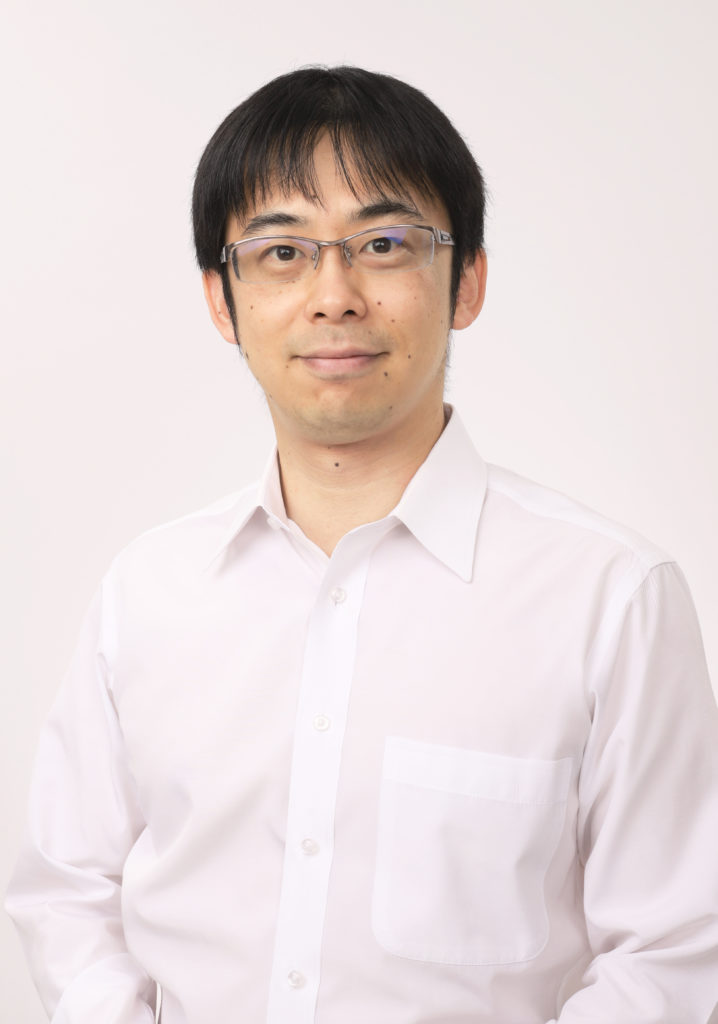
In a recent project, we explored how the arrangement and frequency of specific language elements (distributional factors) influence the accuracy of L2 learners’ use of inflectional morphemes (e.g., -ed, -ing) in their writing. Our research delved into the impact of the following three factors:
- Frequency of inflected forms: We investigated whether the frequency of an inflected form in learners’ input affects its accuracy. For example, if learners encounter ‘asked’ more often than ‘graduated’, do they use ‘asked’ more accurately?
- Proportional frequency of inflected forms: We examined whether the proportional occurrence of an inflected form affects its accuracy of use. To illustrate, if ‘arrived’ makes up a large proportion of all instances of ‘arrive’, ‘arrives’, ‘arrived’, and ‘arriving’, while ‘liked’ makes up a smaller proportion in the ‘like’, ‘likes’, ‘liked’, and ‘liking’ group, do learners use ‘arrived’ more accurately than ‘liked’?
- Formulaicity: We explored whether formulaicity, or the extent to which a given word sequence is a fixed, prefabricated, or memorised expression, influences its accuracy of use. For instance, does the more predictable context of ‘graduated’ in ‘I graduated from college’ lead to more accurate use than the less predictable ‘wanted’ in ‘wanted a lot of’?
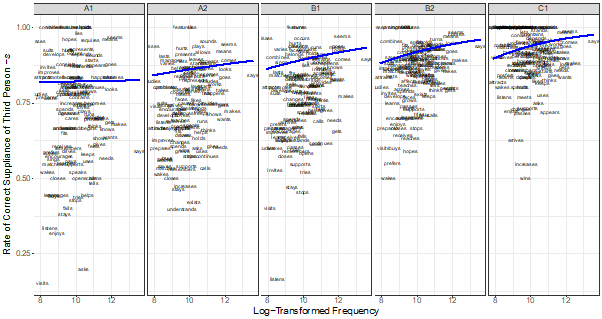
In this project, I employed BlueBEAR’s HPC service for two primary tasks. First, I utilised its power to compute the distributional factors within a large-scale corpus of American English that served as a proxy for L2 learners’ input. For instance, in calculating formulaicity, I needed to tally the frequency of a broad array of three- to five-word sequences with an open slot, such as ‘with all ___ of’, where any word could be used to fill in the blank. This sort of operation, which creates a frequency list of four-word combinations from a large-scale corpus and counts the frequency of such gapped word sequences, is more manageable using the computational resources of BlueBEAR’s HPC service. It offers memory far larger than that of an average desktop computer, making these intricate computations straightforward and efficient.
because we could run multiple jobs in parallel on BlueBEAR’s HPC, we significantly reduced the required computation time.
Second, the substantial memory capacity of BlueBEAR’s HPC was also indispensable for the Bayesian statistical modelling used in our project. As in many other areas of the social sciences, Bayesian analyses are becoming increasingly popular in our field of research. However, estimating parameters using Markov chain Monte Carlo methods—often employed in Bayesian statistics—can be computationally demanding. Without the substantial memory provided by BlueBEAR, it might not have been possible to estimate the parameters of the hierarchical Bayesian model used in our project.
In addition, BlueBEAR enabled us to partially parallelise the computation process. Combined with earlier text processing, the entire computation could have taken over two weeks to complete. However, because we could run multiple jobs in parallel on BlueBEAR’s HPC, we significantly reduced the required computation time.
Thus, the large memory and parallel-processing capabilities offered by BlueBEAR’s HPC service have been instrumental in my research, making tasks that would otherwise be extraordinarily time-consuming or even impossible quite manageable and efficient.
We were so pleased to hear of how Akira was able to make use of what is on offer from Advanced Research Computing, particularly to hear of how he has made use of BlueBEAR HPC, its many cores and large memory nodes – if you have any examples of how it has helped your research then do get in contact with us at bearinfo@contacts.bham.ac.uk. We are always looking for good examples of use of High Performance Computing to nominate for HPC Wire Awards – see our recent winners for more details.