In this case study we hear from Daniel Galvis, a researcher in the Institute of Metabolism and Systems Research, who has been making use of BlueBEAR to enable his research into the role that network structure and node-intrinsic heterogeneities play in coordinating complex behaviours.
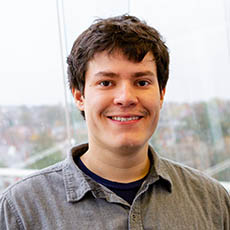
My name is Daniel Galvis and I am a postdoctoral researcher in the Centre for Systems Modelling and Quantitative Biomedicine (SMQB) at UoB.
My primary area of interest is studying the role that network structure and node-intrinsic heterogeneities play in coordinating complex behaviours. Through a combination of data analysis and mathematical modelling, I study the role that these features play in maintaining healthy dynamics within interconnected systems (e.g., brain areas, pancreatic islets) and the perturbations that predict disease (e.g., epilepsy, diabetes).
Trying to understand the role that some feature of a network plays in governing how it will act can be challenging. For example, we may observe statistical differences in brain networks that predict whether a person will be at increased risk of having seizures. But, can we also say something about why these changes have that effect? A major hurdle is that complex networks are appropriately named, and a difference in some network feature could reflect a whole host of different perturbations to a network. Features like small-worldness or degree distribution do not uniquely define the structure of a network, and there could be a functionally infinite number of ways to arrange a network with those same features.
Luckily, the computational power available through BEAR has enabled me to jump this hurdle by employing a class of computational methods called Monte-Carlo experiments. In simple terms, a Monte Carlo experiment is a computer-based method where we run a simulation many times with random inputs to understand the distribution of possible outcomes for a given problem. In my case, I can randomly produce many networks that all have the same feature, simulate dynamics on each of those networks, and quantify the effect that changes in the feature will have statistically on the resulting activity of the network.
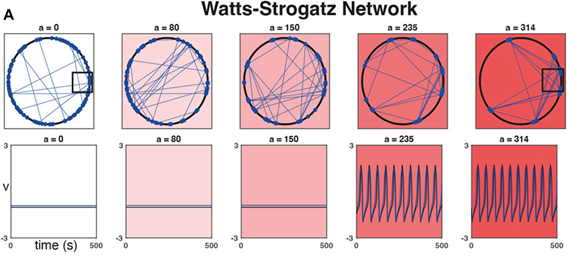
As a practical example, we were inspired by studies looking at the importance of heterogeneity and cytoarchitecture in governing healthy glucose metabolism by the pancreas. We developed a measure, called sortedness, for the likelihood of cells with similar excitability (i.e., responsiveness to an input like glucose) to form connections with one another. We also developed an algorithm for producing networks with desired sortedness. The top panels of Fig., 1 A and B show networks with fixed connectivity structure (small-world ring and 3-D cluster, respectively) but increasing levels of this sortedness (i.e., blue nodes begin clustering). The bottom panels show that changes in sortedness can cause the network to become active and produce oscillations without having to change the input strength (G). Fig. 1C shows that for these two network structures, increasing the sortedness of the network leads to a decrease in the threshold input required to produce a response.
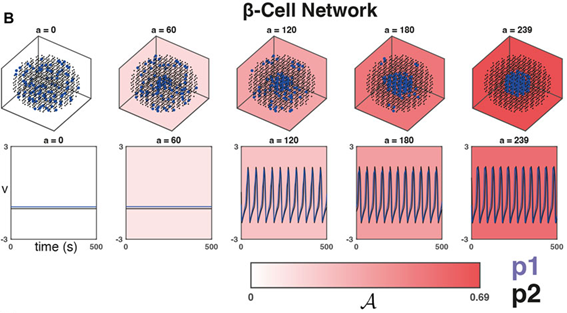
This was an interesting result, but we needed to prove that these results hold more generally. For one thing, given a fixed connectivity structure, there are still many ways to arrange heterogeneous nodes to achieve a desired sortedness. Perhaps more importantly, there are also many ways to arrange network connections and produce a structure with the same small-worldness. The Watts-Strogatz model (WS) was developed to randomly generate graphs with this desired feature (Fig., 1A is an example). Empowered by the resources available through BEAR and employing these Monte-Carlo methods, and with a little help from Gaussian process regression, we then repeated the experiment on many WS networks and many nodal arrangements of varying sortedness. Through this, we confirmed that increased sortedness of high-excitability cells reliably predicts a lower threshold (w.r.t. G) for producing a network-wide response. If you are interested and would like to know more, please see [1].
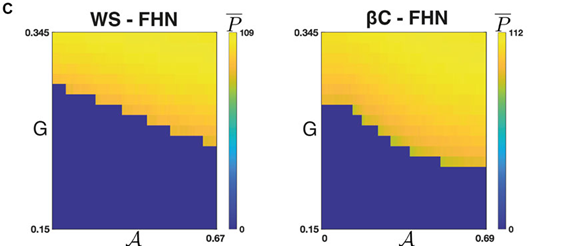
[1] https://www.frontiersin.org/articles/10.3389/fnetp.2023.1170930/full
[2] https://royalsocietypublishing.org/doi/full/10.1098/rsif.2021.0925
[3] https://www.nature.com/articles/s41467-022-28640-x
[4] https://www.birmingham.ac.uk/research/systems-modelling-and-quantitative-biomedicine/seedcorn-projects/round-3
We were so pleased to hear of how Daniel was able to make use of what is on offer from Advanced Research Computing, particularly to hear of how he has made use of BlueBEAR HPC and its many cores – if you have any examples of how it has helped your research then do get in contact with us at bearinfo@contacts.bham.ac.uk. We are always looking for good examples of use of High Performance Computing to nominate for HPC Wire Awards – see our recent winners for more details.