In this case study, we hear from Jordan Deakin a Ph.D. student in Psychology, who has been making use of BlueBEAR and the BEAR Research Data Store to enable her research into visual selective attention.
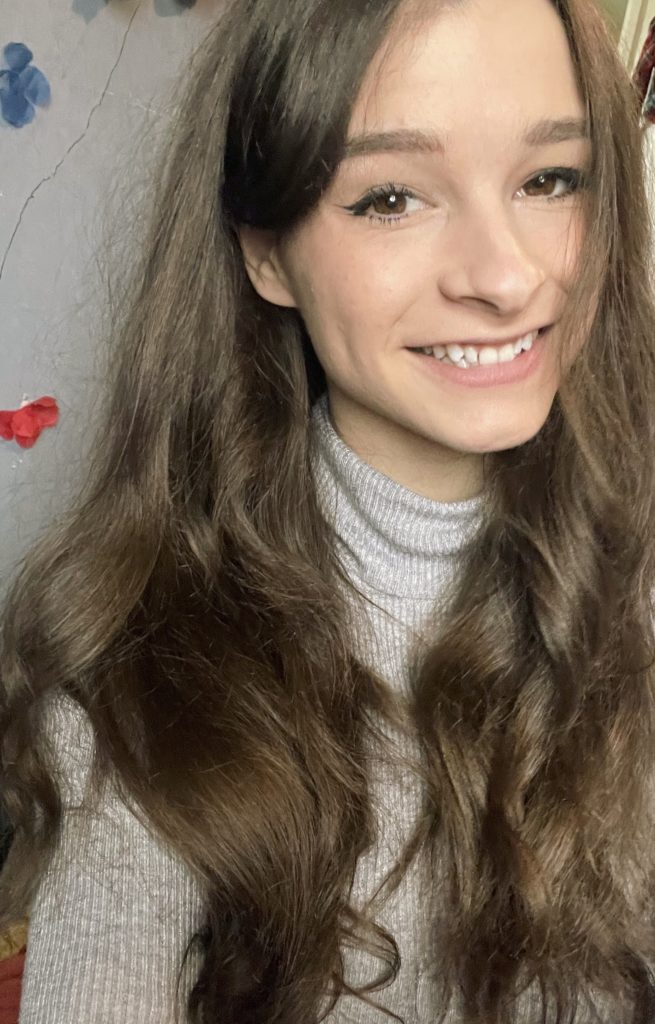
My PhD project explores how visual selective attention enables us to focus on task relevant information while filtering out interference. Typical investigations have used simple mean reaction times and error rates, comparing conditions with and without interference. However, there exists models which incorporate entire reaction time and accuracy distributions and by fitting a model to data, the model can give a much clearer insight into how people suppress interference from distracting stimuli, beyond average reaction time.
The issue is that fitting a model can be very computationally expensive, especially when using Bayesian inference like we do. The algorithms I use for fitting and comparing models (Differential Evolution Markov Chain Monte Carlo, DE-MCMC and Thermodynamic Integration for DE-MCMC, (TIDE)) involve a process of thousands of iterations in which the model is repeatedly simulated and compared to the data. To assess quality of fit, a likelihood function is required. However, for the models I work with, the likelihood function is computationally intractable meaning it must be approximated with kernel density estimation. Again, this approximation requires an iterative process which takes a very long time. On my personal laptop, fitting a model to data from one participant can take weeks. Now, imagine having data for over 200 participants and two models which need to be compared. As you can imagine, such a project is simply not feasible if you don’t have the right kit.
With BEAR’s HPC resources, I can fit models to full datasets in a matter of hours.
At the start of my PhD, I was excited to dive into the world of model fitting, before quickly realising just how computationally expensive model fitting can be. However, one day my brilliant supervisor Dr. Dietmar Heinke introduced me to BEAR. With BEAR’s HPC resources, I can fit models to full datasets in a matter of hours. Not only is the model fitting procedure extremely quick now, but BEAR’s slurm capabilities allow me to run multiple participants in parallel through array jobs. This has allowed me to turn waiting times of months into hours and my project would simply be impossible without it. Not only this but with thousands of iterations, data files very quickly become huge! However, BEAR’s Research Data Store (RDS) gives me more than enough space to store this data and the regular backing up of data by the RDS has saved my PhD more than once after I’ve accidentally deleted an important folder or file.
This (BEAR) has allowed me to turn waiting times of months into hours and my project would simply be impossible without it.
In the figure below, I show the fitting of two models of attention (coloured lines) to real human data averaged across subjects (grey dots) using BlueBear. The first figure shows the defective cumulative response time distribution functions (DCDFs) for conditions with and without interference, the second figure shows the difference between the two conditions, the third figure shows the error rates, and the fourth figure shows conditional accuracy functions which show how accuracy changes depending on response time. As the figure shows, BEAR has allowed me to compare these models, showing that the second model (the Dual-Stage Two Phase Model, DSTP, Huebner et al, 2010) clearly fits my data better. With these results, I can explain how and why this model is superior to better understand how selective attention operates in my task.
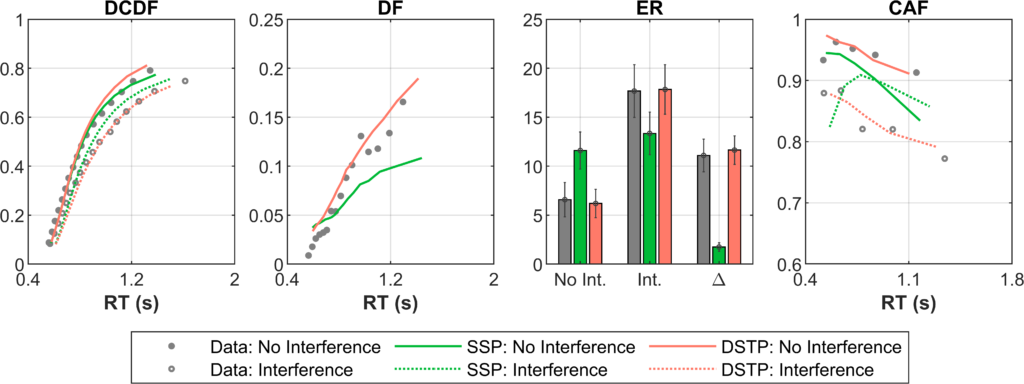
We were so pleased to hear of how Jordan is able to make use of what is on offer from Advanced Research Computing, particularly to hear of how he has made use of BlueBEAR HPC and BEAR RDS – if you have any examples of how it has helped your research then do get in contact with us at bearinfo@contacts.bham.ac.uk. We are always looking for good examples of use of High Performance Computing to nominate for HPC Wire Awards – see our recent winners for more details.